How AI and MDM work together to drive business success
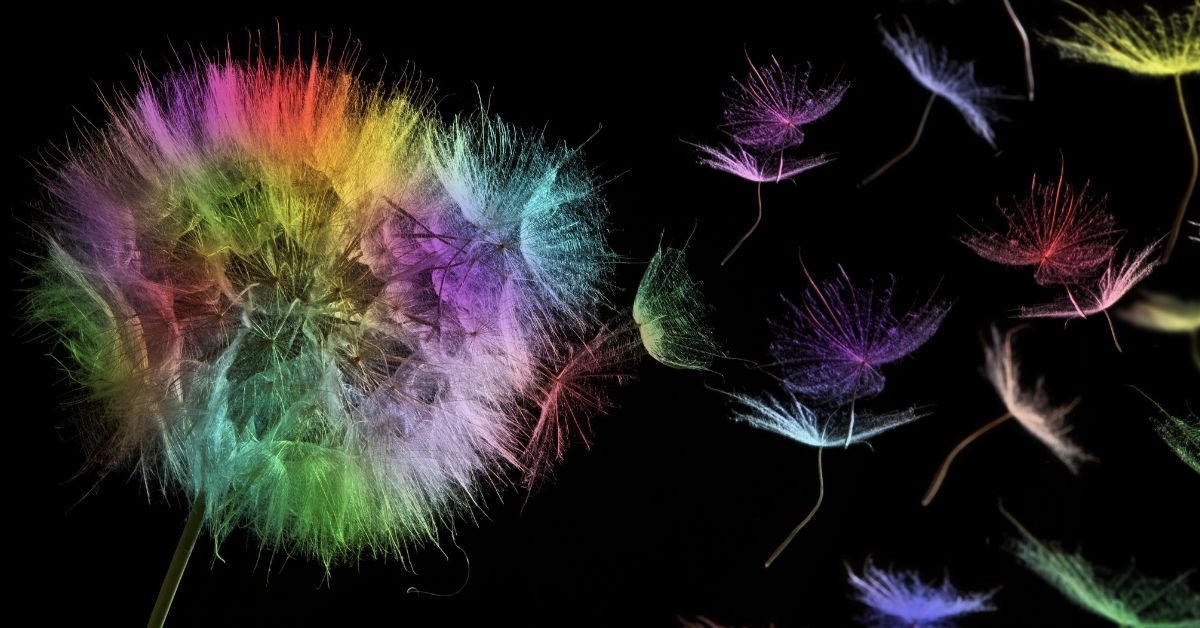
In today's fast-paced world, Artificial Intelligence (AI) is becoming a must-have for making smart decisions, automating tasks, and discovering hidden insights. But here's the thing: AI is only as brilliant as the data it learns from. That's where Master Data Management (MDM) steps in. Think of MDM as the foundation that ensures your AI uses data that's accurate, consistent, and well-organized.
Now, AI is also changing the MDM game itself! It's automating tasks, boosting data quality, and delivering smarter analytics.
Generative AI has really put data in the spotlight. Unlike the old days where data was collected for specific reports or processes, AI needs a broader, more flexible diet. It craves large, diverse datasets, often mixing internal and external sources. This means it's time to rethink your data management approach to make sure your AI investments actually pay off.
Want to stay ahead? Understanding how AI and MDM work together is key. Let's dive into how AI enhances MDM, how MDM powers AI, and what you can do to leverage this powerful combination.
How AI supports master data management
AI brings new possibilities to MDM, making data management easier and more efficient. One of the biggest challenges in MDM is dealing with messy, inconsistent data. AI can help by spotting errors, fixing inaccuracies, and ensuring standard formats across datasets. It also removes duplicate records by identifying similarities, making sure businesses aren’t working with redundant or conflicting information.
Beyond cleaning up data, AI is a game-changer for compliance and governance. With increasing regulations like GDPR and the EU Data Act, companies must be diligent about how they handle sensitive data. AI streamlines compliance by running automated checks, flagging potential risks, and organizing data in a way that minimizes unauthorized access. This automation saves companies time and ensures they meet regulatory requirements without relying on manual oversight.
Another area where AI excels is in categorizing and labeling data. Traditionally, businesses had to manually tag large volumes of data, a process that was time-consuming and prone to human error. AI simplifies this by analyzing text, images, and other unstructured data to apply metadata and categorize information efficiently. As a result, companies can retrieve and use data more effectively.
AI also plays a significant role in providing real-time insights. Businesses no longer have to rely solely on historical reports to guide their decisions. AI can analyze data in real-time, identifying trends, predicting future outcomes, and uncovering hidden patterns. This helps organizations react more quickly to market changes, streamline operations, and gain a competitive edge.
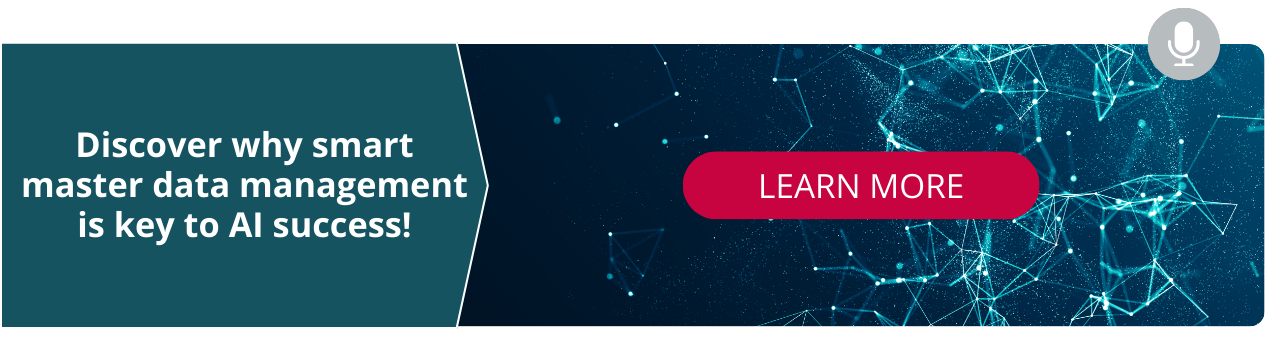
How master data management supports AI
While AI enhances MDM, good data management is just as essential for AI to function properly. AI models need reliable data to perform well, and MDM ensures that data is clean, structured, and free from inconsistencies. Without high-quality data, AI models can produce flawed or biased results, leading to poor business decisions. MDM also prevents outdated or incorrect information from corrupting AI-driven insights.
Another critical function of MDM is breaking down data silos. AI thrives when it has access to a variety of data sources, but many organizations struggle with fragmented data across different departments. MDM bridges these gaps by integrating internal and external data, making it easier for AI to generate a complete and accurate picture of the business landscape. Companies that invest in MDM are better positioned to use AI effectively across departments, from marketing to supply chain management.
MDM also builds trust in AI-generated insights. Many businesses hesitate to adopt AI because they lack visibility into how decisions are made. MDM provides clear data lineage, allowing businesses to trace where their AI models are pulling information from. This transparency fosters confidence in AI-driven recommendations and helps organizations implement AI with greater assurance.
In a nutshell:
1. Ensuring high-quality data for AI models
AI models are only as good as the data they are trained on. MDM helps by:
- Providing clean, structured, and reliable data.
- Eliminating inconsistencies that could lead to biased or inaccurate AI predictions.
- Maintaining a single source of truth, which enhances AI-driven analytics.
- Learn how CDQ ensures high data quality for you
2. Facilitating data integration across systems
AI often requires data from multiple sources to deliver meaningful insights. MDM enables:
- Seamless integration of internal and external data.
- Cross-functional collaboration by breaking down data silos.
- Enhanced interoperability between different business units.
- Learn how CDQ supports data integration
3. Enhancing AI explainability and trust
AI adoption is often hindered by concerns over transparency and trust. MDM provides:
- Clear data lineage to track where AI-generated insights originate.
- Better visibility into how AI models use enterprise data.
- Increased confidence in AI-driven decision-making processes.
- Learn how CDQ delivers more trust
Overcoming challenges: best practices for AI-driven MDM
Ready to make AI and MDM work together? Here's what you need:
First, you need a solid data strategy. Define how AI fits into your overall data management plan and make sure your data governance frameworks are up to the task.
Second, invest in the right tools. Your AI and MDM solutions should support both structured and unstructured data, giving you the flexibility you need.
Third, encourage collaboration. AI and MDM initiatives can't live in silos. Data needs to be shared across teams to get the full picture. Overcome internal barriers and create a culture of data-sharing to improve AI's accuracy and business impact.
Fourth, always prioritize regulatory compliance. As AI-driven data initiatives become more sophisticated, staying compliant with regulations is essential. Proactively monitor your data policies and ensure that AI is being used ethically and responsibly.
Finally, continuously monitor and improve data quality. AI is not a set-it-and-forget-it solution. It requires ongoing maintenance and refinement. Regular audits, updates, and feedback loops will help ensure that AI models remain accurate and aligned with your business objectives.
To wrap it up:
- Develop a clear data strategy: Define the role of AI in your data governance framework.
- Invest in scalable AI and MDM tools: Ensure your technology stack can support both structured and unstructured data.
- Foster cross-department collaboration: Encourage business units to share data and align AI initiatives with MDM goals.
- Implement robust data governance: Establish data ownership, compliance, and security measures to mitigate risks.
- Continuously monitor and improve data quality: Use AI-powered analytics to identify areas for data enhancement.
What happens next?
The combination of AI and Master Data Management is a game-changer. AI makes data management more efficient, while MDM ensures that AI models work with reliable, high-quality data. Together, they help you make smarter decisions, improve operations, and stay competitive.
But remember, AI is only as good as the data it learns from. If your data is messy, outdated, or locked in silos, AI's potential will be wasted. That's why you need to prioritize data management - cleaning, organizing, and governing your data so that AI can provide meaningful insights.
So, what's your next step? Take a close look at your data strategy, identify gaps, and make sure your data isn't holding back your AI ambitions. The organizations that invest in high-quality data management today will be the ones leading AI-driven innovation tomorrow.
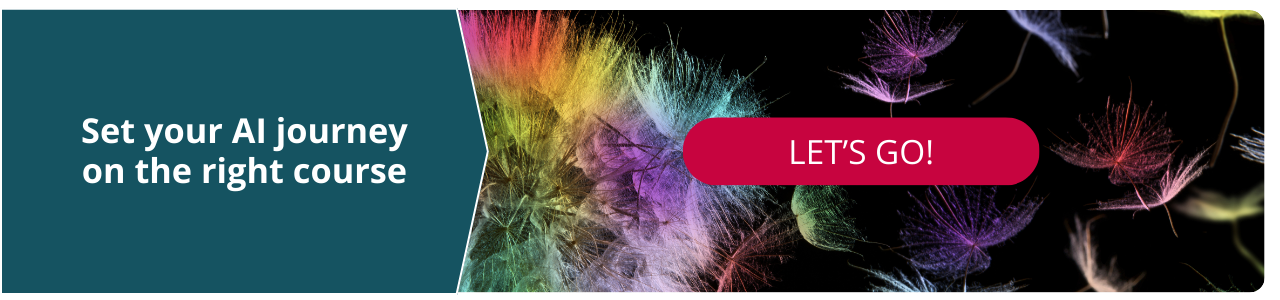
Get our monthly e-mail!
Related blogs
Master Data Management meets AI: work smarter, not harder
Let's be honest, master data management (MDM) often feels like a Sisyphean task. Wrangling inconsistent data, battling data decay, and striving for a single…
Trust, automation, and the future of master data management
Master data management (MDM) is a vital, albeit often underappreciated, foundation of modern business operations. When done right, it ensures smooth processes,…