Data sharing at its finest: CDQ Data Sharing Community
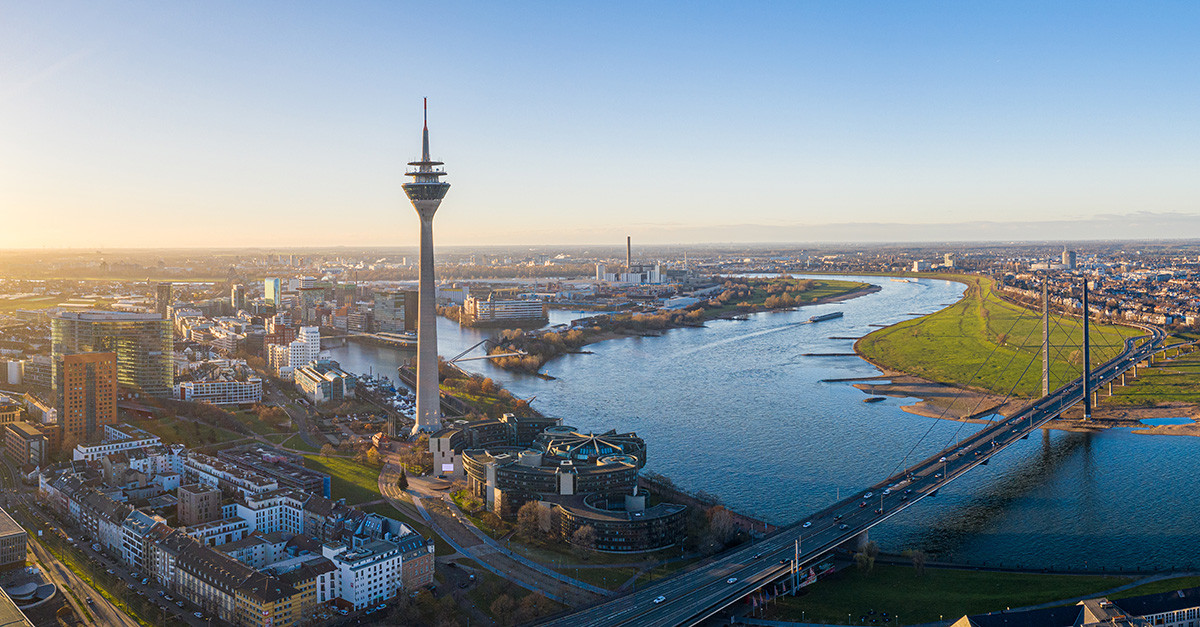
Step into the world of master data management with our CDQ Data Sharing Community workshop, held on April 19-20 in Düsseldorf. Over two invigorating days, 45 participants on-site and 45 data enthusiasts online embarked on a deep dive into the realm of master data.
Yet again, data management industry leaders, experts, and practitioners came together to explore the transformative potential of data sharing. Over two days, participants openly shared their experiences, interacted with peers, asked thought-provoking questions, and engaged in discussions on good practices and enhancing CDQ data solutions based on valuable community feedback.
The workshop witnessed the participation of 30 member companies, including 5 new community members, all of whom demonstrated a shared commitment to harnessing the power of data for organizational growth and success. The agenda was thoughtfully crafted to showcase various implementations of CDQ solutions and concrete use cases, enabling attendees to learn from different perspectives and gain practical insights that could be applied to their own data strategies.
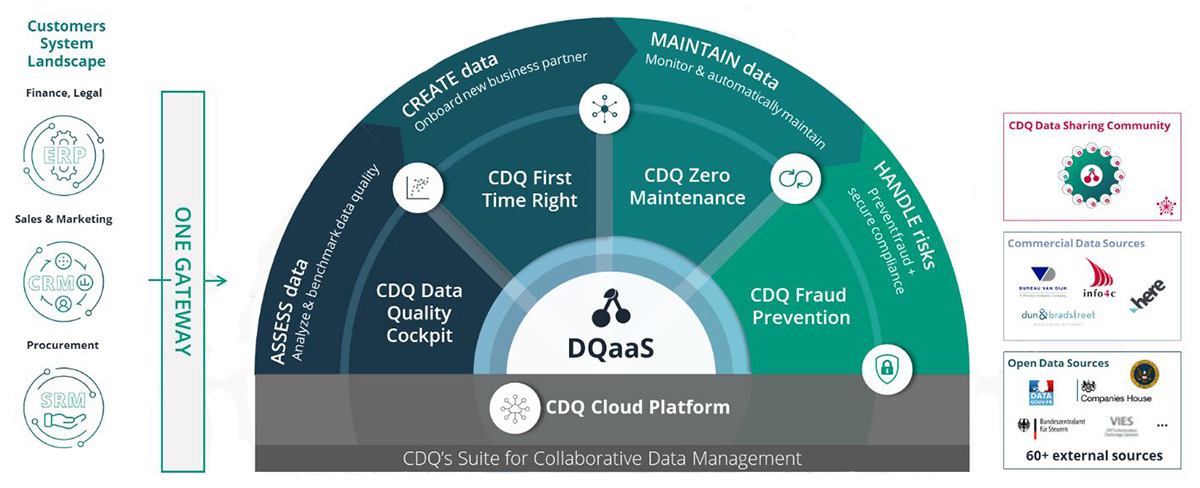
Assessing data with Rheinmetall
Rheinmetall's presentation at the workshop shed light on their relatable journey towards data transformation. They faced numerous challenges, including a high volume of legacy data spanning back to the 90s, a lack of duplicate prevention measures across ERP systems, absence of archiving activities for master data, constantly increasing storage needs, and no defined data ownership structure.
To achieve their vision of transitioning to S/4HANA with a solid foundation of cleansed data, Rheinmetall implemented a structured approach. They first focused on defining roles, responsibilities, and communication channels, establishing a role glossary to ensure clarity and understanding. Tasks, roles, and systems were aligned, fostering effective communication within the organization.
Rheinmetall then embarked on a two-phase journey to achieve their targets. In the "Get-clean" phase, outdated legacy data related to creditors and debtors was thoroughly cleaned. The goal was to avoid carrying unnecessary data into the new system, identify and eliminate duplicates, and verify the accuracy of data content. This process was an integral part of their migration concepts for transformation to S/4HANA.
Building upon this foundation, Rheinmetall initiated the "Stay-clean" phase, which focused on maintaining data quality to ensure continuous high standards. This phase included ongoing activities such as duplicate checks, data enrichment, and harmonization efforts. The central team worked in collaboration with divisions to implement this phase, supporting the organization's harmonization and standardization processes.
Visit CDQ Data Quality Cockpit
Looking for even more efficiency and value, Rheinmetall embarks on additional projects beyond their initial duplicate data cleansing efforts. These projects include providing support for carve-in/carve-out (M&A) scenarios, optimizing material master data for high-bay warehouse space, and leveraging their strong network within Rheinmetall for further initiatives.
Rheinmetall's presentation served as an authentic narrative of their journey, showcasing the challenges they faced and the structured approach they implemented to achieve their vision. Their commitment to data quality and efficiency has not only laid a solid foundation for their transition to S/4HANA but also empowered them to embark on additional projects that create value across the organization.
Community user group “Data quality”
A comprehensive overview of user group activities to enhance data quality assessment through collaboration within the community addressed core topics that formed the foundation of their initiatives.
With established Data Quality Community Rules and defined responsibilities and ownership of different topics within the community, members are able to identify specific areas where they could contribute their expertise and take ownership, fostering a sense of collective responsibility for data quality improvement.
To streamline the process, Data quality user group highlighted the importance of collecting and executing feedback from the community. This feedback plays a crucial role in shaping the direction of CDQ initiatives and ensures smooth collaboration and knowledge transfer within the community. By fostering a sense of shared responsibility, streamlining processes, and leveraging community feedback, this sub-community drives positive change in the data quality landscape, creating a more robust and efficient environment for all Data Sharing Community members.
Creating data with Tetra Pak and Covestro
CDQ First Time Right solution was a focal point for Tetra Pak and Covestro, who showcased their implementations and best practices. CDQ's First Time Right solution offers a range of capabilities such as duplicate search, external data source integration, address cleansing and enrichment, rule-based data quality checks, and tax identifier verification.
Tetra Pak
Tetra Pak shared their case study on the SAP MDG-CDQ Integration Project, highlighting the significant benefits they achieved through this initiative.
The first major benefit Tetra Pak experienced was a reduction in the time spent creating new customer data. By integrating SAP Master Data Governance with CDQ, they streamlined the process and enhanced efficiency in customer data creation.
Another notable achievement was the reduction in the number of MDG change requests required to maintain existing customer data. This optimization not only saved time but also improved overall data management practices within the organization.
Tetra Pak emphasized the improvement in data quality for customers, which was made possible through the introduction of CDQ Data Validation Rules. By implementing these rules, they ensured that data entered for customers adhered to predefined validation criteria, resulting in enhanced data accuracy and reliability.
The organization also showcased their implementation of Business Data Validation rule management by country. This approach enabled them to tailor data validation rules specific to each country, ensuring compliance and data quality on a global scale.
Watch our webinar with Tetra Pak to see their system demo!
Covestro
Covestro has successfully implemented several CDQ integration initiatives, delivering tangible benefits to their master data management processes. Since November 2022, they have been actively utilizing the Bank Data Trust Score Check in SAP MDG, which has enhanced the accuracy and reliability of their bank data validation.
Another successful integration effort is the Weekly Duplicate Matching - this feature enables them to identify and eliminate duplicate data entries, ensuring data consistency and reducing the risk of errors. On top of that, Covestro implemented Historical Duplicate Matching, further enhancing their data quality by eliminating duplicate records from historical data sets. This step contributes to maintaining clean and reliable data across their systems.
Covestro's ongoing efforts include the Address Data Lookup & Curation, which was in the quality assurance testing phase during the presentation.. By ensuring the accuracy and consistency of address data, Covestro aims to improve their overall data quality and enhance operational efficiency.
Covestro also emphasized the benefits of CDQ Apps and API integrations for their master data:
- reduced efforts for vendor authenticity checks
- higher first-time right rates through address lookup and data derivations
- more efficient duplicate cleansing
- automated error corrections
- reduction in fraud risks.
Maintaining data with Siemens
The next part of the workshop focused on CDQ Zero Maintenance solution and recommendations for its implementation within the Data Sharing Community. The session included insights from Siemens on their perspective and the integration of CDQ capabilities.
CDQ Zero Maintenance Solution offers powerful capabilities that allow companies to automatically receive customer-relevant data updates from the CDQ data sharing pool and external data sources. It encompasses company-specific updates, address and business partner curation, and the identification of duplicate records.
Siemens presented their approach to automation of updates from trusted data sources and outlined their mission statement for business partner data. They emphasized the significance of accurate Business Partner Master Data for efficient and integrated business processes. Siemens Corporate Master Data Services manages a vast amount of Business Partner Master Data and aims to ensure high-quality data by cross-checking and enriching the information.
Siemens described their approach to Business Partner Enrichment and Validation during the Get Clean Phase. This includes conducting high-level matching of Business Partner Master Data and enhancing business partners with missing identifiers such as trade/commercial registration numbers, tax identification numbers, LEI, and other identifiers. The goal is to ensure comprehensive and accurate data.
In addition to business partner enrichment, Siemens focused on the importance of business partner monitoring. By implementing state-of-the-art data sharing and monitoring capabilities, they can maintain a high level of data quality and detect changes to attributes of external business partners early on.
For Business Partner Monitoring, Siemens aims to detect changes to business partners at an early stage. This includes changes in legal status, insolvencies, mergers and acquisitions, carve-outs of business partners, updates to registered addresses, and other relevant updates. Swift communication of these changes is crucial to maintain data integrity.
Handling risks with Dovista
Dovista presented their approach to incorporating CDQ First Time Right and CDQ Compliance Screening with their Master Data Simplified (it.mds) solution, aiming to streamline compliance and MDM requirements. The goal was to have one front-end system that controls data and compliance across the entire Dovista organization.
To achieve this, Dovista implemented a common MDM front-end, which allows them to manage their master data objects through a centralized platform. They partnered with CDQ to simplify their master data processes and incorporate compliance checks, rules, and processes with built-in sanction screening capabilities.
Visit CDQ Compliance Screening
By integrating CDQ's solutions behind the scenes, Dovista was able to retrospectively distribute data and establish one source of truth for their various ERP platforms. This ensured real-time data availability and promoted a First Time Right approach, reducing errors and improving data quality.
In preparation for the implementation, Dovista focused on documentation and education. They established 19 core business processes and created process flow diagrams that define the activities and functionalities supported by CDQ. Each process was documented in a separate How-to Guide, providing step-by-step instructions.
Roles and responsibilities were assigned based on functions and user roles, and workflow management was implemented accordingly. This allowed for clear accountability and efficient collaboration within the organization.
The symbiosis between it.mds and CDQ provided Dovista with a robust solution that harmonized their compliance screenings and master data management. By leveraging the capabilities of CDQ, Dovista achieved greater data accuracy, reduced duplicate entries, implemented sanction screenings, and conducted bank data checks. The integration of these functionalities within their MDM processes enabled a seamless and efficient data management experience.
Dovista's presentation showcased the successful collaboration between it.mds and CDQ, highlighting the benefits of their symbiotic relationship in streamlining compliance and MDM processes for improved data quality and operational effectiveness.
Watch our webinar with NTT Business Solutions to see how it.mds works!
Add-on: Custom Data Quality Rules Extension
Custom Data Quality Rules Extension enhances the benefits of CDQ's validation service by making it more company-specific. This add-on allows for the inclusion of additional data fields and business logics, resulting in improved data quality and more tailored validation processes.
By extending the data quality validation, the add-on enables automation and efficiency improvements in various aspects of data management. The data maintenance process can be further automated, leading to increased speed and efficiency in data maintenance tasks. The data quality reporting and dashboard can be more specific, allowing for better monitoring and improvement of company-specific data quality. Additionally, the data creation process can be automated, enhancing the speed and efficiency of data creation. Furthermore, the add-on allows for the specification and combination of risk validations or indicators, further reducing risks in data management processes.
Bayer’s experience with custom data quality rules
To showcase the power of custom data quality rules extension, success story of Bayer proved how more automation leads to less processing time and less workload.
- Speed: The approval process for standard low-risk requests was significantly accelerated. Simple changes that previously took 2 days according to service level agreements are now processed in just 2 seconds through auto-approvals. This improvement has made the source-to-pay workflow more fluent and efficient.
- Trust: The automation rate increased by 40%. Leveraging their extensive and mature data governance knowledge, Bayer implemented data quality rules that reliably automate standard change requests, enhancing the trust in the data management processes.
- Efficiency: The processing time was reduced by 50%. On average, the time taken for processing change requests in MDG decreased from 28 hours to 14 hours, from the start of the process to approval. This significant reduction in processing time contributes to overall operational efficiency.
Bayer's presentation demonstrated the tangible benefits of implementing custom data quality rules, including improved speed, increased automation, and enhanced efficiency in data management processes.
The community members' discussions around the add-on highlighted how this extension can enhance CDQ's validation service and enable organizations to tailor their data quality processes to their specific needs, resulting in improved data quality, more speed, increased automation, and greater operational efficiency.
Thank you!
The workshop was filled with thought-provoking discussions and presentations from industry experts who shared their insights and best practices on the importance of data sharing. IThe level of engagement and the quality of the discussions that took place during the conference were truly impressive.
We already look forward to the next Data Sharing Community workshop in September!
Will you join us?
Get our e-mail!
Related blogs
Unlocking the power of Data Sharing: the CDQ approach
For nearly a decade, CDQ has been leading the way, pioneering a secure and collaborative approach to business partner data sharing. With our innovative Data…
From e-bikes to e-mail guards: CDQ Data Sharing Community Workshop highlights
Another unforgettable CDQ Data Sharing Community Workshop has wrapped up, and what an incredible two days it’s been! Packed with insightful sessions, engaging…
Why collaborative location management is the future (and why AI isn’t the answer)
We’ve all heard the saying: “If all you have is a hammer, everything looks like a nail.” These days, AI feels like that hammer -an exciting, powerful tool being…