AI and MDM: Engineering Data Excellence
Unlock the Potential of Artificial Intelligence with Trusted Data Quality
... high-quality data remains the foundation for success
increase spending on GenAI
in their organizations
as the greatest challenge to
realize AI potential
claim their organizations
lack the right data foundation
by Thomas H. Davenport, Randy Bean, and Richard Wang
Reliable and Bias-Free AI
Master Data Management Best Practices for AI success
Just as the typical “garbage in, garbage out” principle, the same applies to AI: if the input data used to train an AI model is inaccurate, incomplete, inconsistent, or biased, the model's predictions and decisions will be too. To reduce project risk and fully leverage the potential of generative AI technology, businesses must prioritize data quality through effective master data management.
Best practices to consider:
data practices, mitigate biases, safeguard privacy,
and compliance with regulatory standards.
duplications, and inconsistencies, maintaining
high data quality.
that measure completeness, accuracy, consistency, and timeliness,
providing a quantitative measure of data reliability.
teams, differentiating sensitive information from data clutter
to implement appropriate controls.
Leveraging AI to Enhance Data Quality
Reliable data is essential for businesses leveraging AI for competitive advantage, such as in customer segmentation and supply chain optimization. But AI isn’t just about using data; it can also improve data quality throughout the entire Data Value Chain.
Data is the foundation of digital business models and operations, and it’s crucial for managing risks and meeting regulatory requirements. The real challenge for decision-makers is to effectively harness data as a valuable resource that drives business success.
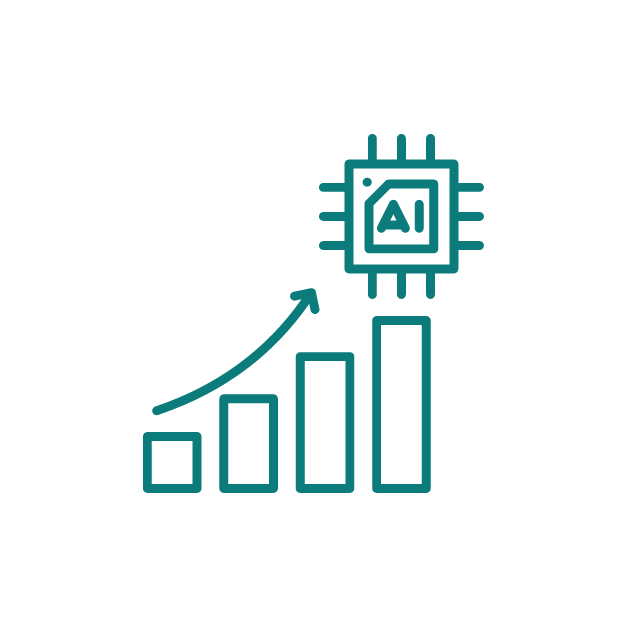
Enhancing Data Quality with AI
Master data cleansing is often a tedious search in countless tables and systems, involving at least three different business processes that need to agree on standards, priorities, responsibilities. And if data cleansing services are considered, most of the time they are not flexible enough to take business-specific requirements and fuzzy logic into consideration.
At CDQ, we harness the power of artificial intelligence to transform and digitize data cleansing. By employing advanced algorithms and machine learning techniques, CDQ automates and refines the process of identifying, correcting, and managing data quality issues.
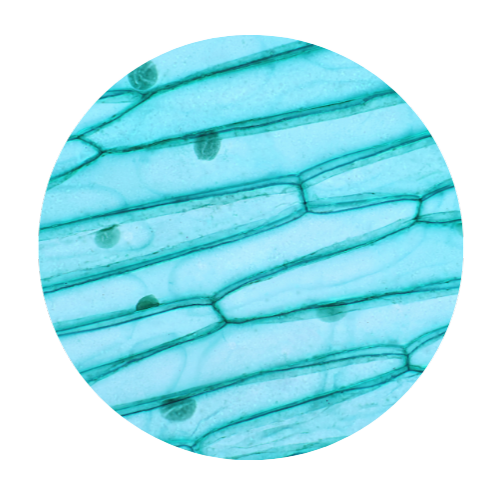
Here’s a closer look at how CDQ uses AI to boost the data cleansing process
Data Profiling and Assessment
CDQ utilizes AI algorithms to identify patterns and anomalies within datasets. This capability enables efficient detection of inconsistencies and duplicates. Furthermore, CDQ employs machine learning models to evaluate data quality using metrics such as completeness, accuracy, consistency, and timeliness.
Duplicate Detection and Merging
By employing AI, CDQ can identify and merge duplicate records by recognizing that different entries refer to the same entity, even if the data is not identical. Machine learning techniques can match similar but not identical records, improving the accuracy of duplicate detection.
Anomaly Detection
For anomaly detection, CDQ employs AI-powered models to identify outliers that indicate data entry errors or unusual patterns. CDQ's machine learning capabilities allow for the analysis of historical data to detect deviations from expected trends, signaling potential data quality issues.
Scalability and Efficiency
One of the significant advantages of CDQ is its ability to handle large datasets efficiently. This makes CDQ ideal for big data environments. By automating repetitive and time-consuming tasks, CDQ frees up human resources, allowing them to focus on more strategic activities.
Automated Data Correction
In the realm of error detection and correction, you can significantly enhance the process by automatically identifying errors like typos, incorrect formats, and invalid entries, where CDQ identifies a need for improvement. Additionally, CDQ ensures uniformity across datasets by standardizing data formats, units, and values.
Data Enrichment
Enriching datasets is another area where CDQ shows its strength. By integrating external data sources, CDQ fills in missing information and enhances data completeness. And with advanced ML-capabilities, CDQ provides a reliable tool to understand and extract relevant information from unstructured data sources.
Monitoring and Improvement
CDQ ensures continuous monitoring of data quality in real-time, providing alerts and automated responses to emerging issues. Through feedback loops, CDQ’s machine learning models learn from past corrections and user feedback, continually enhancing their accuracy and effectiveness.
By leveraging these AI capabilities, CDQ significantly enhances the accuracy, reliability, and usability of corporate data. This leads to better decision-making and operational efficiency, ultimately driving greater value for the organization.
AI-supported Duplicate Detection
Sartorius was looking for a sustainable solution to mitigate duplicate-related risks: not only ensuring that every data defect could be addressed post-creation, but also onboarding clean, unique records into the system at the first instance.
Duplicate checks are seamlessly integrated into Sartorius system, running automatically in the background. The algorithm swiftly identifies potential duplicates, triggering a streamlined process. When a potential duplicate is detected, a work item is generated for manual review, ensuring accuracy and precision.
You might also like
CDQ and SAP: the master data automation dream team
In the fast-evolving realm of enterprise data management, few aspects are as critical as business partner master data. Whether dealing with customers, suppliers…
Augmenting business partners into trusted Golden Records
Let's explore a fundamental concept that plays a pivotal role in ensuring the integrity and consistency of your business partner information – the golden record…
Trusted Business Partner Data in the Age of AI
High-quality business partner data is the backbone of enterprise success in today's digital landscape. It's about having consistent, up-to-date information on…